“I’d rather be vaguely right, than precisely wrong”
-John Maynard Keynes
We think this phrase from the notable 20th century economist and mathematician helps highlight the basis of informed decision making. Survival instincts learned over millennia affect and bias our decision making. While these biases can be both useful or lead to mistakes, they will always kick in when high value decisions are called for, so understanding these biases is critical. Experience shows that when the stakes are particularly high, the pursuit of precision often invites inaction.
Commodities Forecasting
It is common knowledge that forecasting commodity pricing is an imprecise business. All forecasts are wrong at some point, but what’s really important is understanding how uncertain we are in a given forecast. If you use a particular forecast report every week, do you expect it to be off by a penny or a dime when predicting three months out? That uncertainty defines the risk involved and, generally speaking, a person’s tolerance for risk decreases as the value of the decision increases (fig 1). This can often lead to bad decisions, or worse, no decision at all.
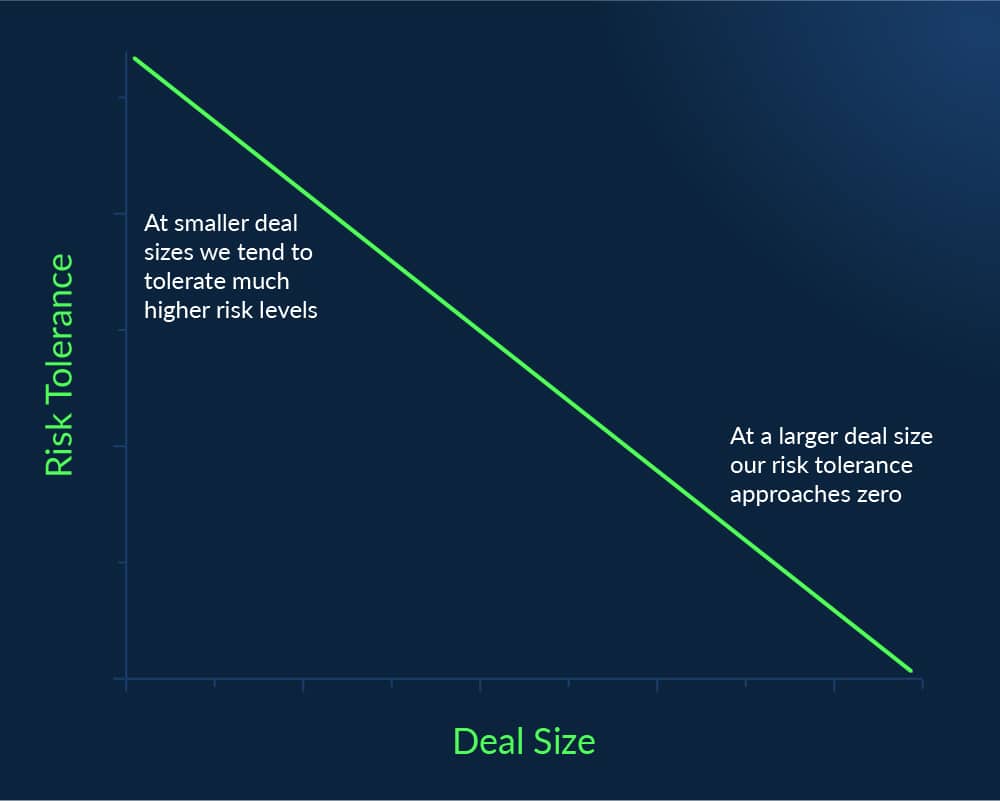
Figure 1. Risk Tolerance vs. Deal Size
Think about the last big decision you made.
You gathered as much information as you could, sorting through news, trends, and historical data, and then the decision was made — yes, no, or not now. While searching for the absolute value of the expected outcome we tend to walk past quantifying the risk involved.
In the graphics below we can see the difference between precision and accuracy. Striving for precision will often give results that are consistent and tightly clustered, but may actually be far from the true market outcome. You can see that the forecasts below are precise, gathered in a tight cluster, lending them credibility, but this is false precision, since they are clustered far from the actual outcome (fig. 2). Consistent and precise, but not particularly accurate.
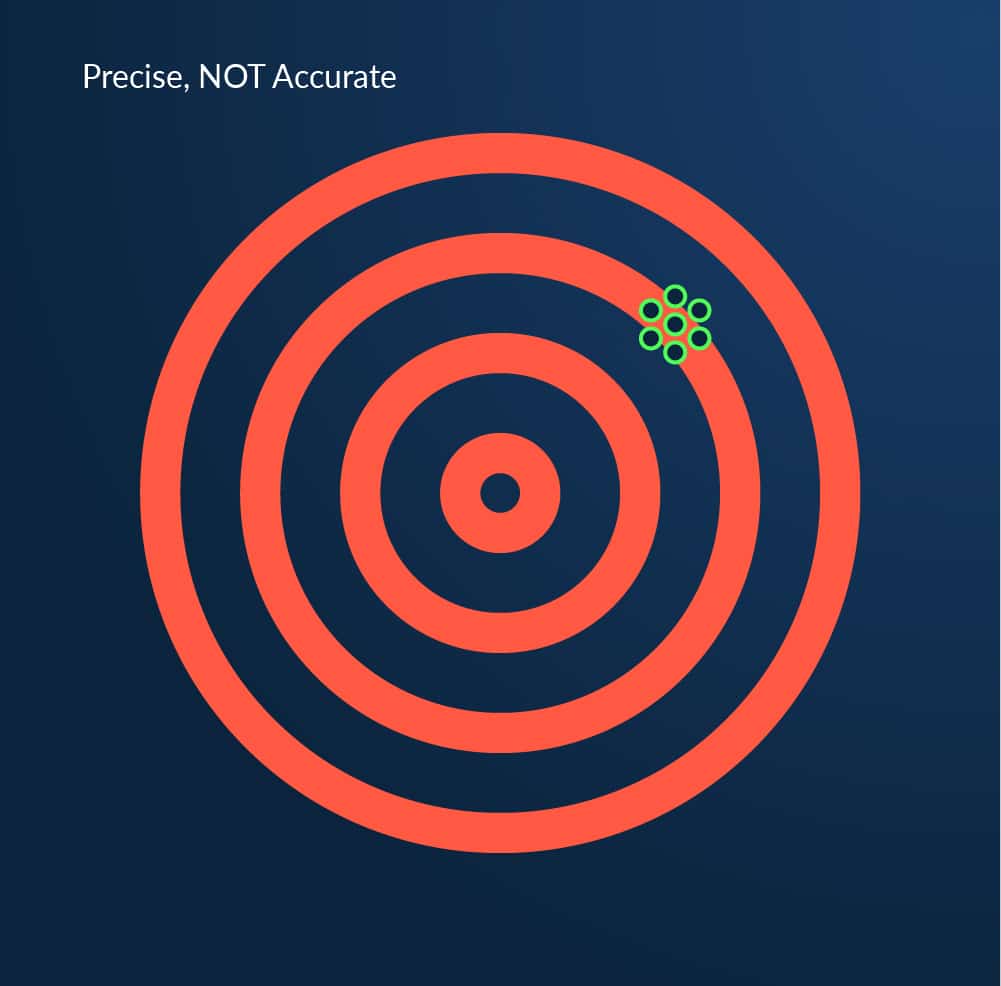
Figure 2. Precise but Not Accurate
Next we see an accurate, but not particularly precise, set of outcomes (fig 3). These relatively accurate results seem to cluster around the 1 to 2 standard deviation range. This means that we are generally in the neighborhood of the right outcome every time. The value over a precisely wrong assumption in this case is 2x to 4x. Thus, striving for precision can result in a costly mistake, or crippling inaction.
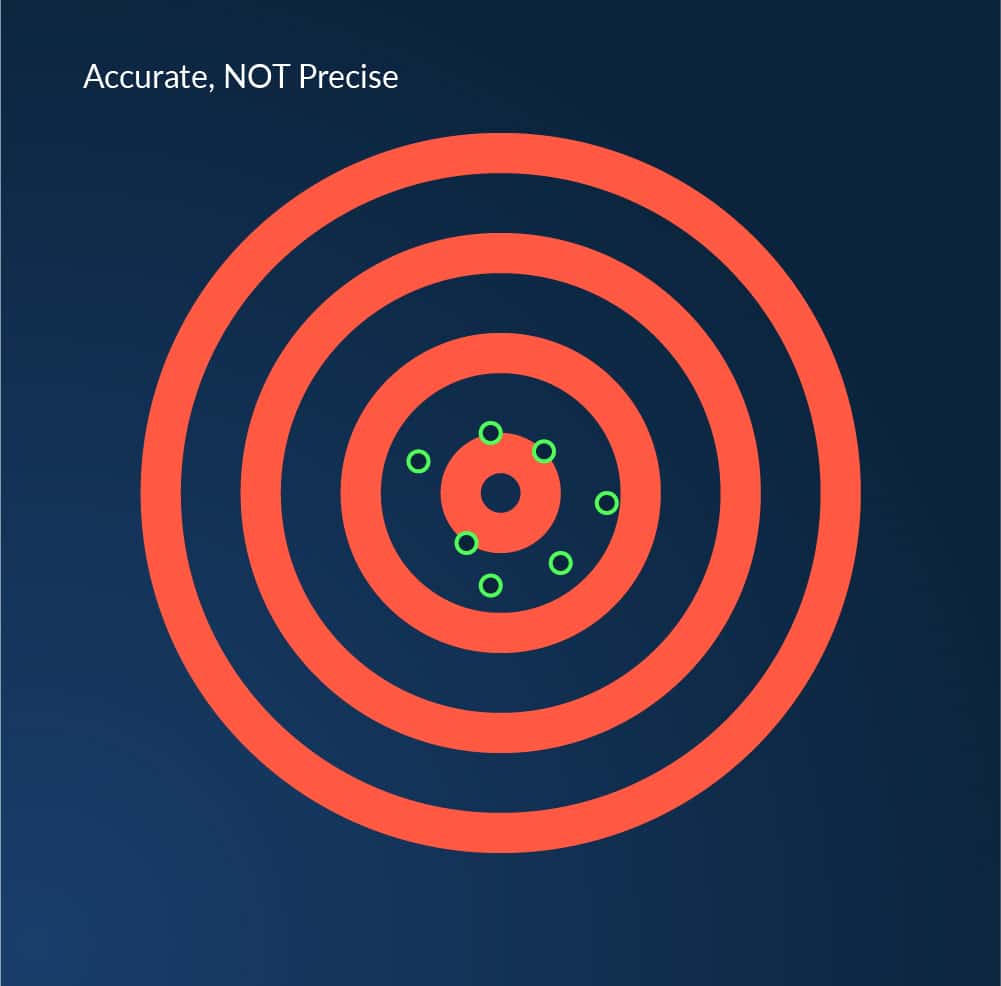
Figure 3. Accurate but Not Precise
Insight into Action
Let’s say that you need to make a choice beef brisket purchasing decision, weeks into the future (fig. 4). You’ve observed that carcass values are going up, so you are inclined to pay attention to the CME futures curve and its impact on brisket pricing. There’s a CME-based ratio forecast model that you may consider. That’s an example of using one, precise point of view.
But what if the history and seasonality of that cut combined with other relevant data tells us that the price should not increase to the extremes of the CME forecast level, creating a second view point? And what if machine learning price forecasting showed us the historical seasonality and price trends, leading to yet a third price forecast.
Finally, what if the choice brisket price ratio to the graded cutout indicates that there is some alignment with the Machine Learning forecast, diverging from the CME forecast we initially believed? We can assess that our two consistent forecast alternatives may very well create a more accurate view of the true beef brisket market, while not being as precise (or tightly clustered) as one singular forecast model.
Figure 4. Choice Beef Brisket – Historical Price with 26 Week Price Forecast
Getting comfortable with taking action (go, or no go) is the key to good decision making and requires a confident understanding of the level of risk involved with any given scenario. The more confident you are in the range of misses (how far off is my forecast likely to be), the easier it is to act decisively. Leveraging experience and tools designed to validate assumptions and highlight the risk involved can help.
Don’t let the pursuit of precision be the reason for leaving money on the table.